Do you ever struggle to keep up with the pace of change in the digital marketing and tech space? That's no surprise, because it's rapid - but we've got you covered.
I'm Robin, Associate Director of Strategy and Innovation at Aira, and I've been wanting to share our thoughts on industry developments, new tech and what we've been working on.
We’ve got some interesting reading to share, a tool we’ve been using at Aira, and thoughts on some of the attribution updates in GA4.
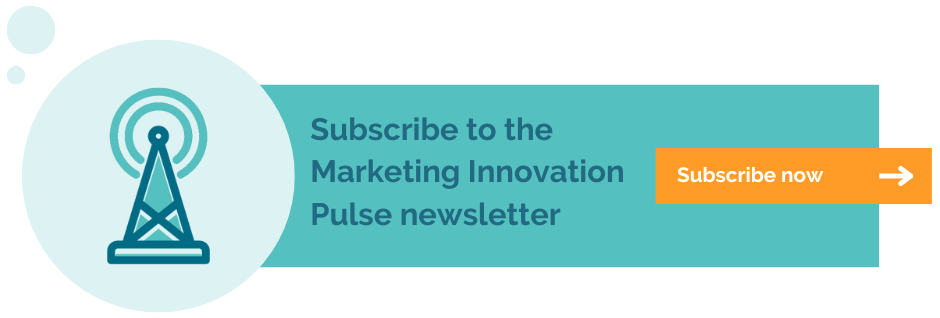
What we’ve been reading
There’s a lot of interesting stuff flying around at the moment. As you might expect a lot of it is related to Machine Learning/GPT-type stuff. This is not going to be all-ML all the time, but this time around we’ll just relax into it and share a bunch of things on that topic.
- 'You Are Not a Parrot' - great article from Intelligencer about the difference between humans and tools like Chat GPT
- Some people are bringing together lots of different LLMs (tools like Chat GPT) to perform more complex tasks. They are messy, cobbled together, and pretty imperfect, but Thomas Smith thinks that in that way they are kind of like the human brain
- Some people think that we should stop training AI systems now, so they signed an open letter calling for a halt to all AI training
- The OpenAI CEO confirming they are not working on GPT-5 and responding to that letter
What we’ve been doing
- You may have seen an announcement from Paddy about Aira’s new approach to content distribution. It might seem like a big shift from the outside, particularly for people who have always known Aira’s Digital PR prowess, but it’s actually a continuation of something we’ve been doing internally for quite some time.
- We shared a tool we’ve been using to generate Title Tags and Meta Descriptions to get past the initial “blank page” stage. That Twitter thread also includes some of Aira’s policies on the role of ML tools in our work.
- Our Paid Media team bagged the UK Paid Media award for best use of Facebook and Instagram ads, and Holly Pierce, one of our Paid Media Consultants won a rising star award.
What we’ve been thinking - GA4 changes and attribution
On 6th April, Ginny Marvin (Google Ads liaison) announced that first-click, linear, position-based and time decay attribution models are being removed from Google Ads and GA4.
Unsurprisingly, this set off a fresh wave of consternation from marketers about having to rely on GA4 come July (seriously, if you haven’t got it set up by now, you need to get cracking).
It’s worth bearing in mind a couple things about this.
GA4 comes with auto BigQuery exports
You would have had to pay hundreds of thousands of pounds for this with UA. If you want, you can use that granular data to recreate your favourite attribution models for comparison.
I strongly recommend switching on the BigQuery exports if you haven’t already, if only because it’ll give you ongoing granular data access stretching back more than the 14 month limit that GA4 imposes. Even if you don’t think you’ll personally be digging into historic data that much, if you’re thinking of doing any impact modelling or forecasting in the future using tools like Prophet or Causal Impact, you’ll want 24 months worth of data at least.
As Google’s Ads Liaison points out, you can also use explorations to investigate user journeys
This isn’t quite the same as having a model baked in from the start that you can easily flick to and see how different channels are performing. You’ll have to dig to get your answers and, to be honest, I think that’s part of the point.
Google can’t have their free analytics tool eagerly disagreeing with their own ads
As we’re all keenly aware, attribution is almost never a straightforward conversation. For something so ‘mathsy’ there are a lot of opinions that fly around - and it’s only getting harder with cookie changes. On the one hand, cutting down support for models probably makes life easier for Google engineers/processing costs. On the other hand, it almost definitely reduces the scenarios where PPC teams are having their impact questioned by other teams or senior stakeholders who have been flicking between the models available in GA.
I don’t think it’s a coincidence that these models are being removed from Google Ads and GA4 at the same time. I also don’t think we need to be wearing too big a tinfoil hat to remark on the fact that the three models that are sticking around (Last Click, Ads-Preferred Last Click, and Data Driven) are either the easiest to measure, the most likely to make ads look good, or the hardest to pick apart.
So, maybe you’re thinking: ‘What should I do about attribution now?’
It may well not be the first time you’ve thought this, what with the aforementioned cookie stuff, Safari’s ever-tightening grip on tracking, growing ad blocker usage, GDPR etc. etc.
There’s also companies like Uber saying they turned off 80% of their ads and saw no impact, articles suggesting that attribution-based analytics is a scam, and a growing buzz from big tech companies like Facebook about how we need to stop “attributing” and start “modelling”.
As you can imagine, these questions and answers are very important to us at Aira. We’re not interested in how to make analytics numbers look good - our focus is business impact. So doing a bunch of activity that just takes credit for conversions that would have happened anyway is not the one.
The problem with user-tracking approaches
If you’re using tools like GA or ad pixels; click tracking, cookies, and fingerprinting all rely on following people every step of the way.
They assume that every conversion is due to something they have recorded (or some combination of things). Because they are so myopically focused on individual user journeys they are naturally bad at spotting broader patterns. They are less likely to pick up when conversions would happen anyway. So, they can split the numbers up for you and give you an idea of what’s working, but they can’t really give you an answer to the question “what did this get me that I wouldn’t have got anyway?”
The problem with maths-based approaches
Tools like Causal Impact, Robyn, and Uber Orbit look at historic data to try to gauge what the actual impact of activity is. Essentially - they try to understand the patterns of your business so well that they can say “when you changed x, shortly afterwards sales were higher than we’d expect, so we think x is responsible”. As a result - they need lots of data because of how well they need to understand those patterns to spot when something is unusual.
That means it can take a while to see what the true results of activity are. It’s pretty time-intensive to pull the data and it’s almost impossible to use these tools to inform granular decision making (like whether a headline is a good one to use).
The trick is to use both user-tracking and maths-based approaches, but for different things
Understandably, no one wants to run the risk of investing heavily in something that actually doesn’t have any impact, but at the same time we can’t spend all our time trying in vain to measure the value of small pieces of activity which we’re pretty sure are a good idea anyway.
Often the right answer with small-scale tactical stuff is relatively easy to spot. If we optimise a page and we start ranking better for our target keywords, that’s probably a good thing. If we start getting more traffic and that traffic is converting, that’s probably a good thing.
It’s usually the larger-scale decisions that are harder to validate. Should we be spending on brand advertising? Is top-of-funnel activity bringing us revenue further down the line?
We probably won’t change our attitude to top-of-funnel each week, but we could well be changing our headlines that fast. So, standard user-tracking probably gives us most of what we need for the small scale optimisations. Maybe we don’t have easy access to first-click models in GA any more, but we can probably use things like user signals to get an idea of if the traffic we’re bringing onto the site seems engaged. At the same time - we can try to measure our bigger investments, and strategies with some of the more maths-based approaches. When it comes to making decisions about bigger investments - we’re more likely to have long-term data, and more likely to have the time to do this kind of analysis. Aside from anything else, we’d be talking about a quarterly commitment rather than a daily one!
As ever, the last piece of the puzzle is for us all to accept that whatever measurement we choose it will almost always be wrong. While we can try our best to make it as accurate as possible, the goal is not to understand the ultimate ROI of every single small thing we do. We can put sensible measurements in place to avoid making silly decisions, but we’re always going to be doing a whole bunch of things at the same time anyway. If our whole plan relies on us just finding the one perfect change that will bring us business success, we’re already in trouble!
Thanks for reading
I hope you enjoyed this combined reading-list-and-ramble! We’re going to try to do more of these in future so if you particularly liked (or loathed) any parts of this please do let us know!